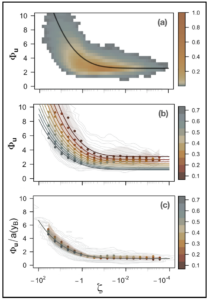
Congratulations to Dr. Marc Calaf! His article with colleague Prof. Stiperski titled “Generalizing Monin-Obukhov Similarity Theory (1954) for Complex Atmospheric Turbulence” is currently featured on the front page of PRL (Physical Review Letters) web page as an Editor’s Suggestion.
Monin-Obukhov Similarity Theory (MOST) is the cornerstone of atmospheric turbulence and forms the basis for parametrizations of turbulent exchange in virtually all numerical models of atmospheric and oceanic flows. Its limitations to flat and horizontally homogeneous terrain and non-scaling of horizontal momentum variances have, however, plagued the theory since its inception. This article presents a first generalized extension of similarity theory universally valid in conditions in which MOST fail. This new breakthrough theory allows a correct representation of turbulence effects in weather, climate, and air-pollution models over the majority of Earth’s surface, thus reducing uncertainties in the surface-atmosphere coupling, especially over particularly vulnerable regions such as polar regions and mountains experiencing unprecedented warming.
You can find a link to the full article here.
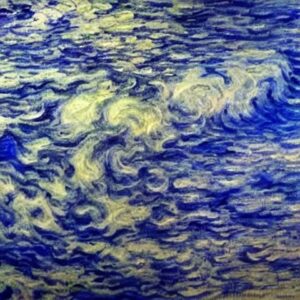